Hyperbolic Active Learning Optimization
HALO introduces a hyperbolic neural network approach to pixel-level active learning for semantic segmentation. We propose the use of epistemic uncertainty as a data acquisition strategy, following the intuition of selecting data points that are the least known. The hyperbolic radius, complemented by prediction entropy, effectively approximates epistemic uncertainty.
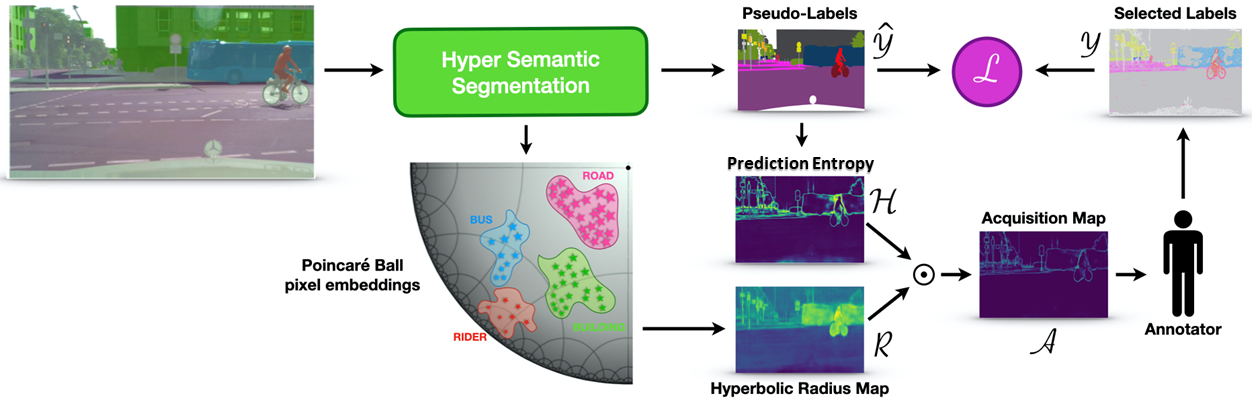
HALO's Breakthrough
HALO sets a new state-of-the-art in active learning for semantic segmentation under domain shift. Key achievements include:
- Novel interpretation of hyperbolic radius as an indicator of data scarcity
- First use of epistemic uncertainty for data acquisition in this context
- Extensive validation on synthetic-to-real benchmarks and adverse weather conditions
- Surpasses supervised domain adaptation performance using only 1% of labels

Try out HALO now!
Experience HALO's power in semantic segmentation!\
Click on the sample images below to see how HALO effectively identifies and labels different elements in the scene, even with limited labeled data.
- The left image represents the semantic segmentation of the scene using only 1% of labeled data.
- The center image shows the hyperbolic radius map produced by the hyperbolic neural network.
- The right image displays the pixels selected by HALO for labeling.